
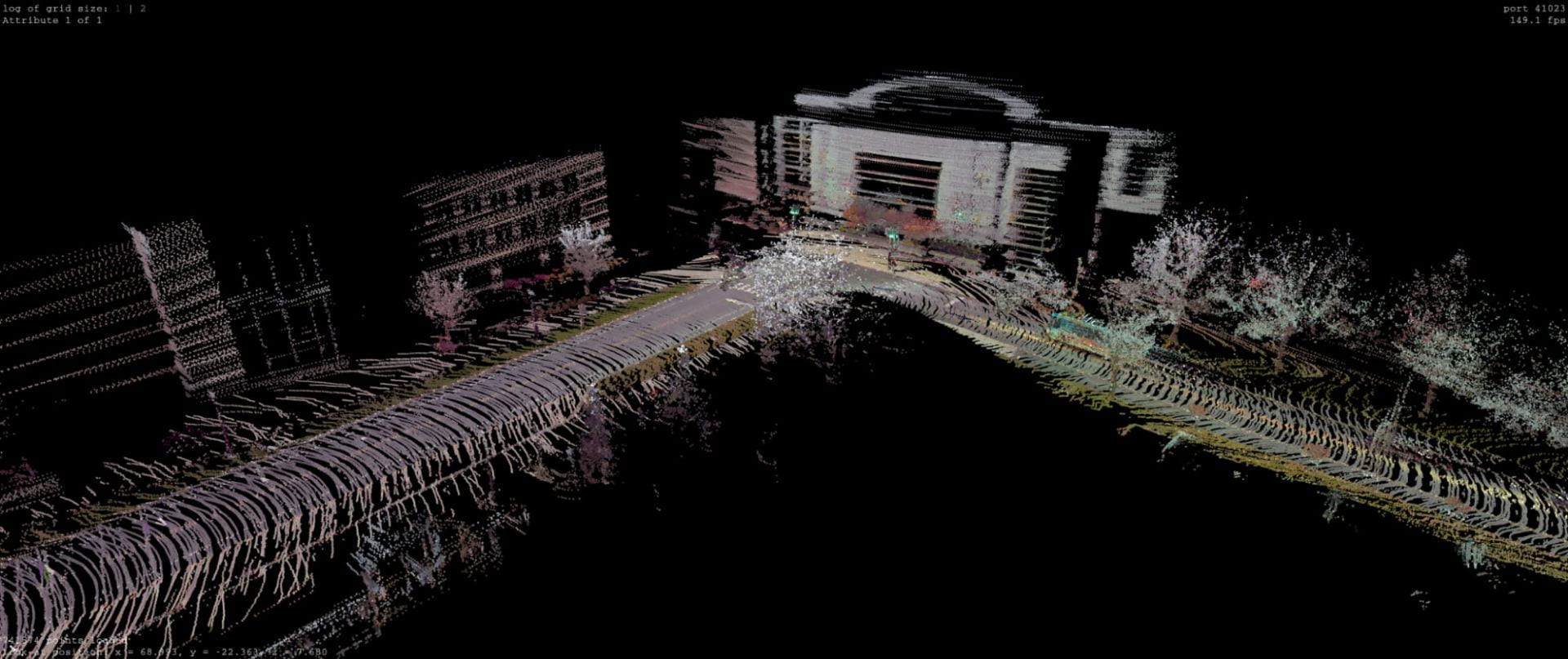
Overview
Advances in perception for self-driving cars have accelerated in recent years due to the availability of large-scale datasets, typically collected at specific locations and under nice weather conditions. Yet, to achieve the high safety requirement, these perceptual systems must operate robustly under a wide variety of weather conditions including snow and rain. In this paper, we present a Ithaca365, a new dataset to enable robust autonomous driving via a novel data collection process — data is repeatedly recorded along a 15 km route under diverse scenes (urban, highway, rural, campus), weather (snow, rain, sun), time (day/night), and traffic conditions (pedestrians, cyclists and cars). The dataset includes images and point clouds from four cameras and LiDAR sensors, along with high-precision GPS/INS to establish correspondence across routes. The dataset includes road and object annotations using amodal masks to capture partial occlusions and 2D/3D bounding boxes. We demonstrate the uniqueness of this dataset by analyzing the performance of baselines in amodal segmentation of road and objects, depth estimation, and 3D object detection. The repeated routes open new research directions in object discovery, continual learning, and anomaly detection.
The Route
A 15 km loop consisting of varying road types and surrounding environments was selected. The route includes university campus, downtown, highway, urban, residential, and rural areas.
Driving was scheduled to capture data at different times of day, including night. Heavy snow situations were captured before and after roads were plowed. A key uniqueness of our dataset is that the same locations can be observed across different weather and time conditions.
Annotations
The dataset includes 7000 frames across 40 collections that include amodal instance, road and lidar labels below.

Amodal Instance annotations
Amodal objects were labeled Scale AI rapid for six foreground object classes: car, bus, truck (includes cargo, fire truck, pickup, ambulance), pedestrians and bicyclist and motorcyclist. There are three main components in this labeling paradigm: first identifying the visible instances of objects, then inferring the occluded instance segmentation mask, and finally labeling the occlusion order for each object. The labeling is performed on the leftmost front facing camera view. We follow the same standard as KINS.
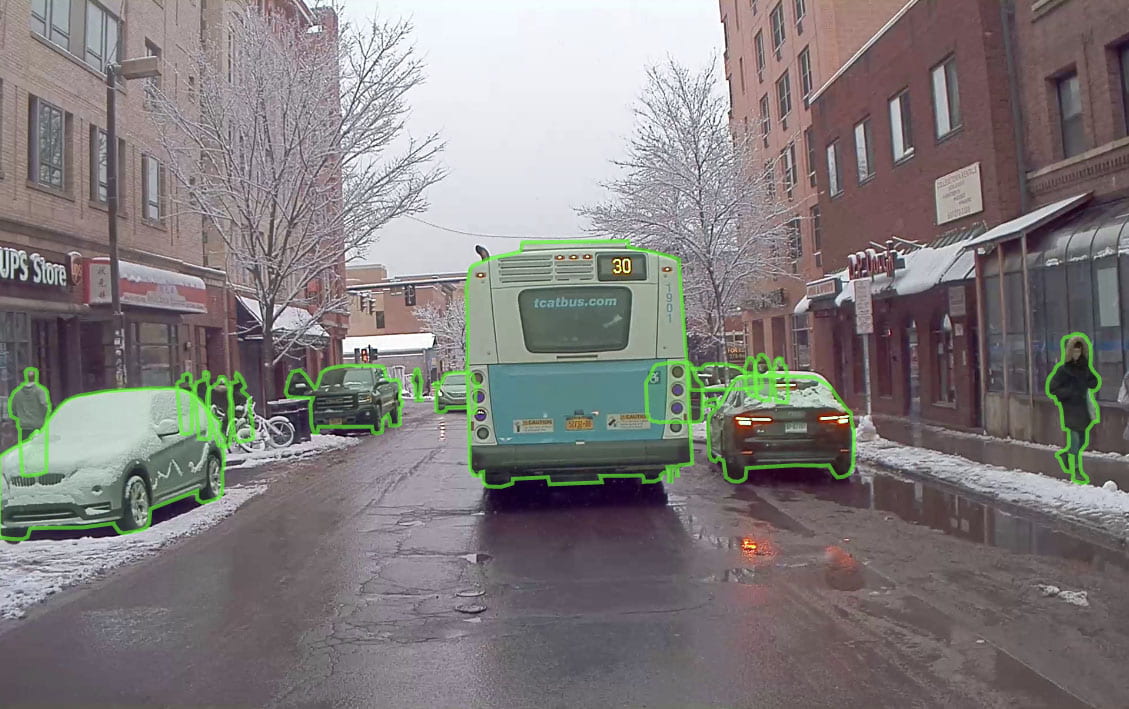
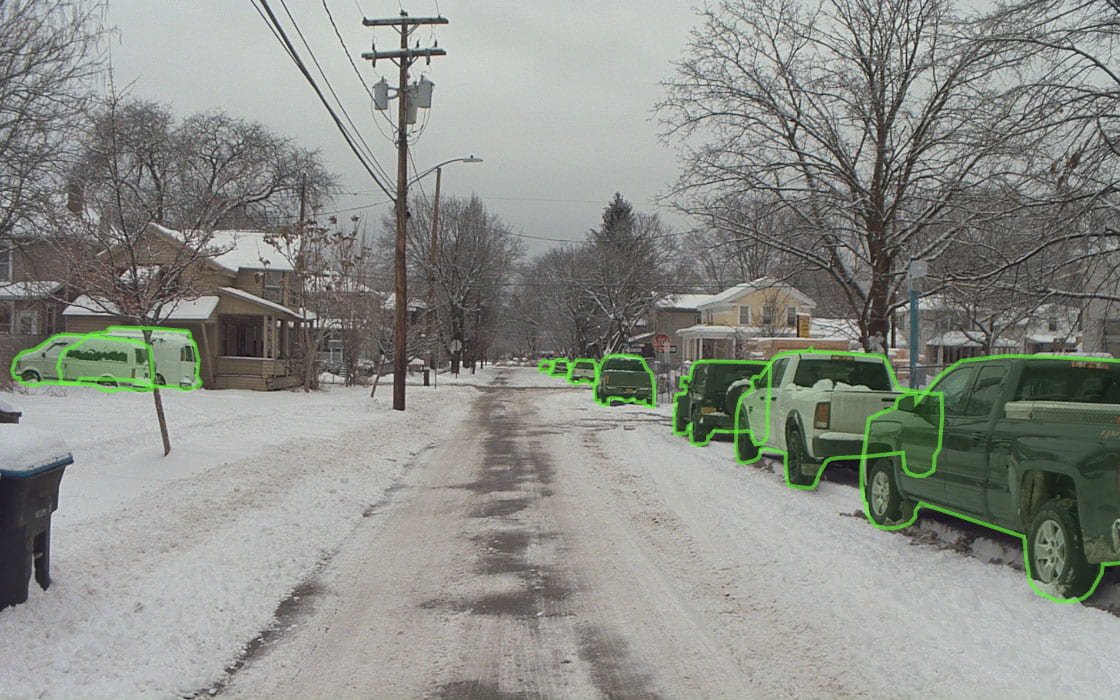
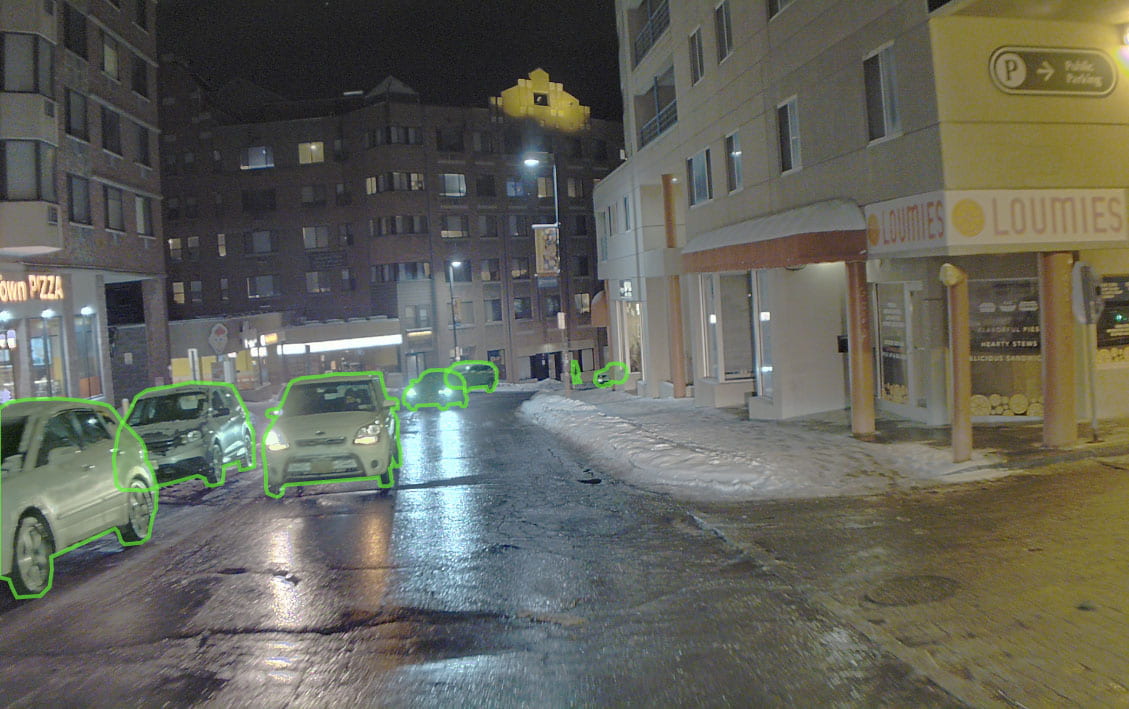
Amodal Road annotations
The route data was divided into 76 intervals. The point clouds were then projected into birds-eye-view (BEV) and the road was labeled using a polygon annotator. Once the road has been labeled in the BEV, which yields the 2D road boundary, the height of the road was determined by decomposing polygons into smaller 150 square meter polygons and fitting a plane to the points within the polygon boundary. The road points are projected to the image and a depth mask is created, obtaining the amodal label for the road.
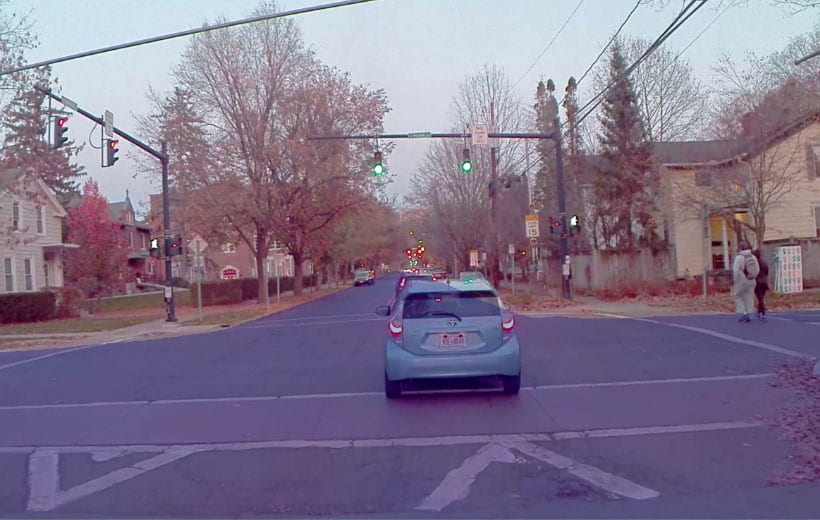
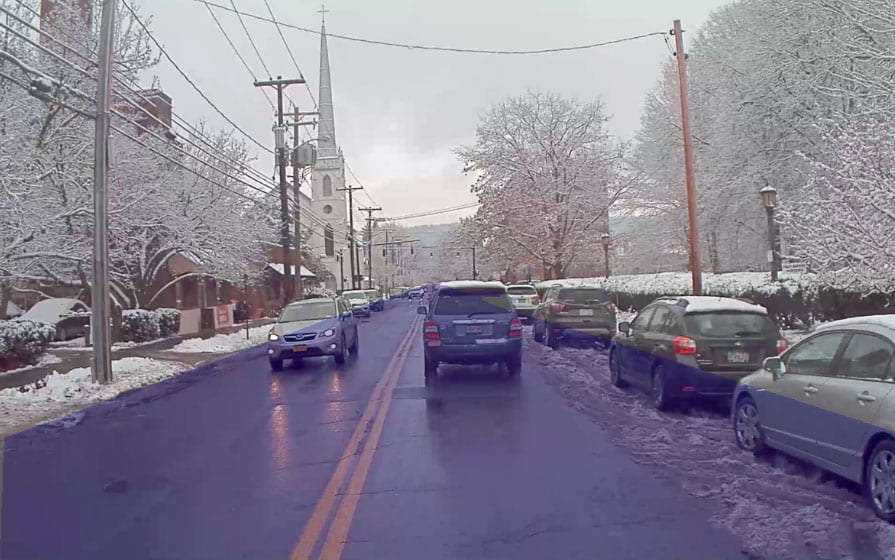
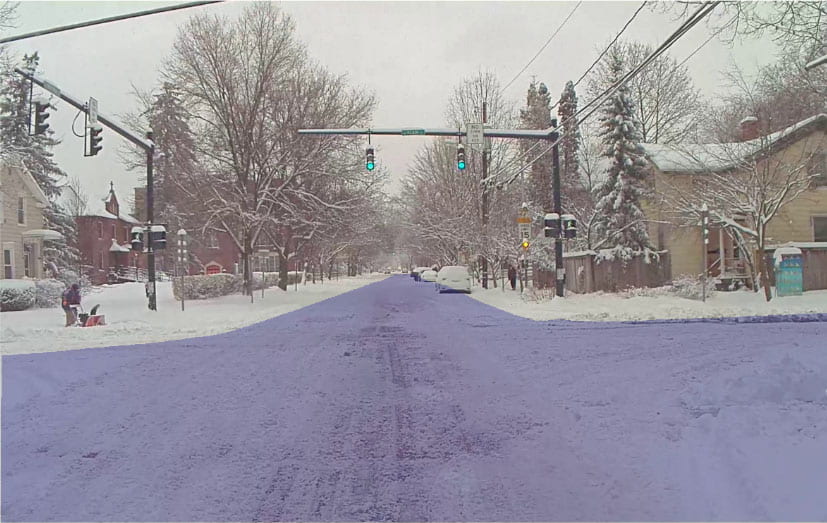
3D Bounding Boxes
Objects were labeled in the lidar pointclouds using Amazon Groung Truth plus service for six object classes: car, bus, truck (includes cargo, fire truck, pickup, ambulance), pedestrians and bicyclist and motorcyclist.
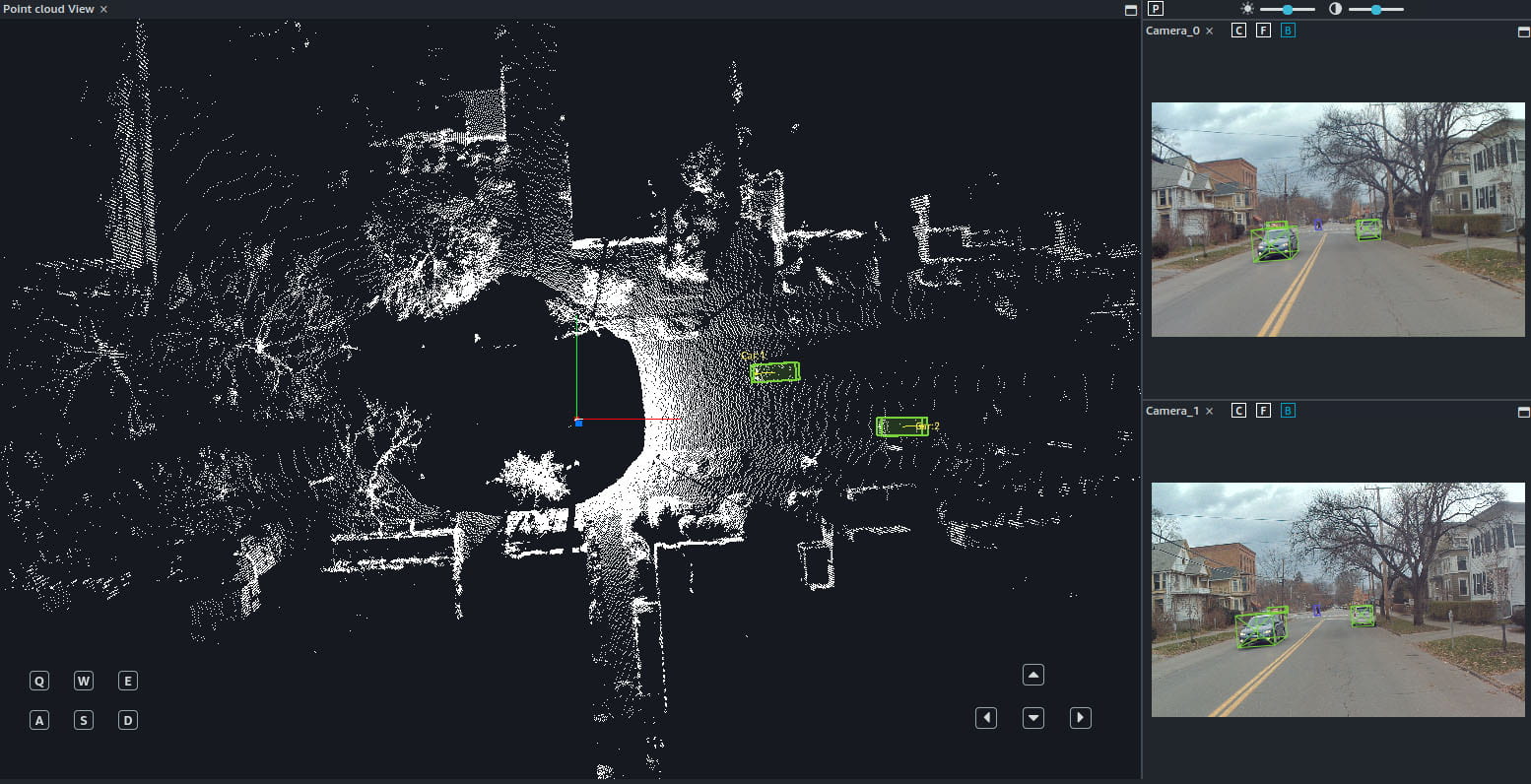
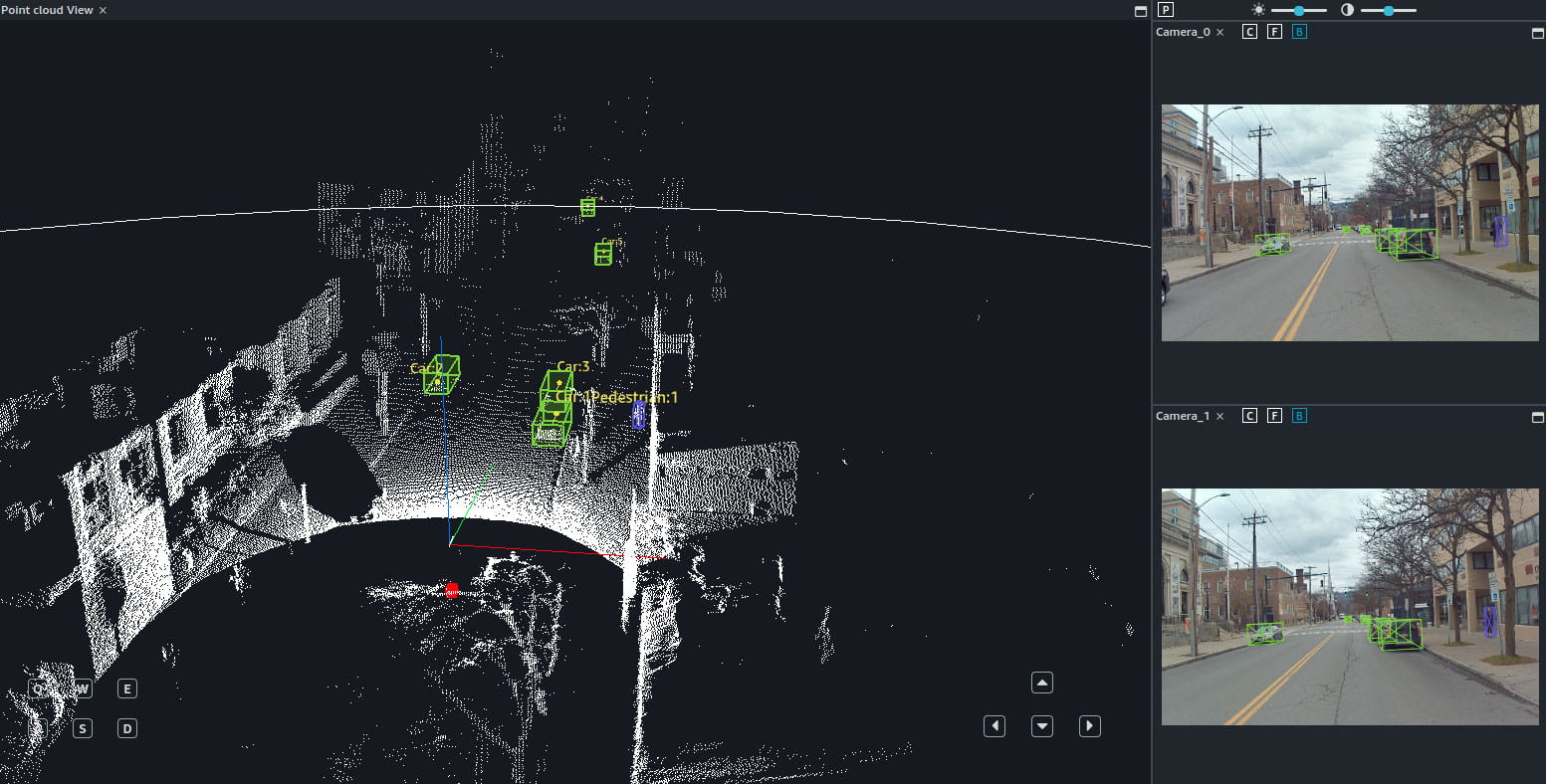